TensorFlow Core tutorial で学んでみた(1) [AI]
TensorFlow の Getting Started With TensorFlow の最初の章、"TensorFlow Core Tutorial" で TensorFlow の基本を学んでみました。

https://www.tensorflow.org/get_started/get_started
まず最初に定数の簡単な例から。コメントが英語なのはご容赦を。
こちらを実行した結果がこちらです。特に説明の必要もなさそうですね。
次に、いわゆる関数を定義します。CやJavaなどと違って行列を変数として与えられることができます。これは便利ですね。
こちらの実行結果がこちらです。
最後に初期値付きの変数を使ったサンプルです。少し小難しいですが差分二乗和を変数と行列でそれぞれで算出しています。reduce_sum という TensorFlow の埋め込み関数も使っています。
こちらを実行してみた結果がこちらです。
ちょっと独特ですが、そんなに難しくなさそうです。次は同じ Core tutorial にある trainAPI について学習してみたいと思います。
(^^)/~




https://www.tensorflow.org/get_started/get_started
まず最初に定数の簡単な例から。コメントが英語なのはご容赦を。
import os os.environ['TF_CPP_MIN_LOG_LEVEL']='2' import tensorflow as tf # define constans node1 = tf.constant(3.0, dtype=tf.float32) node2 = tf.constant(4.0) # execute add sess = tf.Session() node3 = tf.add(node1, node2) print("node3:", node3) print("sess.run(node3):", sess.run(node3))
こちらを実行した結果がこちらです。特に説明の必要もなさそうですね。
C:\Users\Taro\TensorFlow>python constant.py node3: Tensor("Add:0", shape=(), dtype=float32) sess.run(node3): 7.0
次に、いわゆる関数を定義します。CやJavaなどと違って行列を変数として与えられることができます。これは便利ですね。
import os os.environ['TF_CPP_MIN_LOG_LEVEL']='2' import tensorflow as tf # set variables a and b a = tf.placeholder(tf.float32) b = tf.placeholder(tf.float32) # define formula adder_node = a + b # create execution instance sess = tf.Session() # execute '3'+'4.5' print(sess.run(adder_node, {a: 3, b: 4.5})) # execute matrix operation [1, 3] + [2, 4] print(sess.run(adder_node, {a: [1, 3], b: [2, 4]})) # define formula add_and_triple = adder_node * 3.0 # execute operation print(sess.run(add_and_triple, {a: 3, b: 4.5})) # execute matrix operation print(sess.run(add_and_triple, {a: [1, 3], b: [2, 4]}))
こちらの実行結果がこちらです。
C:\Users\Taro\TensorFlow>python placeholder.py 7.5 [ 3. 7.] 22.5 [ 9. 21.]
最後に初期値付きの変数を使ったサンプルです。少し小難しいですが差分二乗和を変数と行列でそれぞれで算出しています。reduce_sum という TensorFlow の埋め込み関数も使っています。
import os os.environ['TF_CPP_MIN_LOG_LEVEL']='2' import tensorflow as tf # define variables with initial value W = tf.Variable([0.3], dtype=tf.float32) b = tf.Variable([-0.3], dtype=tf.float32) # define variable x = tf.placeholder(tf.float32) # define formula linear_model = W * x + b # create exection instance sess = tf.Session() # initialize variables init = tf.global_variables_initializer() sess.run(init) # execute linear_model print(sess.run(linear_model, {x: [1, 2, 3, 4]})) # define complex formura: # squared_deltas = (W * x + b) - y) * ((W * x + b) - y ) # loss = SUM(squared_deltas) y = tf.placeholder(tf.float32) squared_deltas = tf.square(linear_model - y) loss = tf.reduce_sum(squared_deltas) # I don't know why "reduce"? # calculate each element [1, 2, 3, 4] by squared_deltas print(sess.run(squared_deltas, {x: [1, 2, 3, 4], y: [0, -1, -2, -3]})) # calculate each element [1, 2, 3, 4] by loss print(sess.run(loss, {x: [1, 2, 3, 4], y: [0, -1, -2, -3]})) # replace values of W, b fixW = tf.assign(W, [-1.0]) fixb = tf.assign(b, [1.0]) sess.run([fixW, fixb]) # reflect the changes print(sess.run(squared_deltas, {x: [1, 2, 3, 4], y: [0, -1, -2, -3]})) print(sess.run(loss, {x: [1, 2, 3, 4], y: [0, -1, -2, -3]}))
こちらを実行してみた結果がこちらです。
C:\Users\Taro\TensorFlow>python variable.py [ 0. 0.30000001 0.60000002 0.90000004] [ 0. 1.68999982 6.75999928 15.21000099] 23.66 [ 0. 0. 0. 0.] 0.0
ちょっと独特ですが、そんなに難しくなさそうです。次は同じ Core tutorial にある trainAPI について学習してみたいと思います。
(^^)/~

ゼロから作るDeep Learning ―Pythonで学ぶディープラーニングの理論と実装
- 作者: 斎藤 康毅
- 出版社/メーカー: オライリージャパン
- 発売日: 2016/09/24
- メディア: 単行本(ソフトカバー)

TensorFlow機械学習クックブック Pythonベースの活用レシピ60+ (impress top gear)
- 作者: Nick McClure
- 出版社/メーカー: インプレス
- 発売日: 2017/08/14
- メディア: 単行本(ソフトカバー)

Machine Learning With Tensorflow
- 作者: Nishant Shukla
- 出版社/メーカー: Manning Pubns Co
- 発売日: 2017/09/30
- メディア: ペーパーバック
TensorFlow をインストールしてみた! [AI]
Deep Learning を勉強するために、TensorFlow を遅ればせながらインストールしてみました。
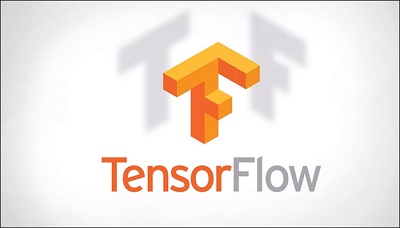
Tensor Flowを動かすには、64bit版 Python が必要なのですが、前回インストールした Python は32bit 版。Pythonをインストールし直しです。バージョンが 3.6 から 3.5 になってしまいますが仕方ありません。
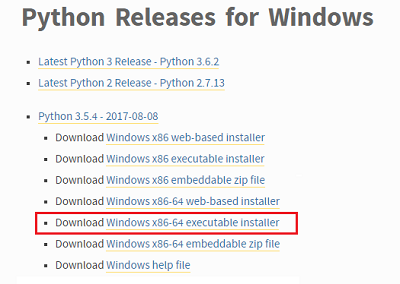
https://www.python.org/downloads/windows/
インストールが終わったら、環境変数にPythonへのパスを通しておきましょう。インストール場所は、Program Filesではないで注意が必要です。
%USERPROFILE%\AppData\Local\Programs\Python\Python35\
%USERPROFILE%\AppData\Local\Programs\Python\Python35\Scripts\
TensorFlow をインストールするのは簡単です。コマンドプロンプトから以下のコマンドを打つだけです。私のノートパソコンはGPUのような大層なものはないので、NonGPU版をインストールします。グラフ描画用の matplotlib もインストールしておきました。
インストールされているか確認をするために動かしてみます。
なんか、変なメッセージが出ましたが動いたようです。今のままだと処理速度が遅いようですね。最適化するには、環境変数におまじないを追加するだけでよさそうです。以下のプログラムでメッセージが出なくなりました。
これで早くなったのかな?スクリプトが短すぎてよく分かりません。
TensorFlow を試してみるには、本家本元のこちらのサイトが一番分かりやすそうです。
Getting Started With TensorFlow
https://www.tensorflow.org/get_started/get_started
さて、環境も整ったので、Deep Learning を学習していこうかな。
(^^)/~



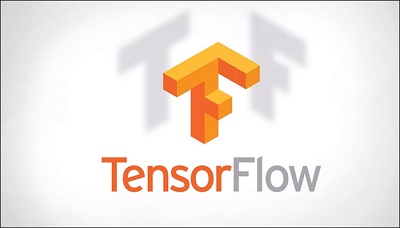
Tensor Flowを動かすには、64bit版 Python が必要なのですが、前回インストールした Python は32bit 版。Pythonをインストールし直しです。バージョンが 3.6 から 3.5 になってしまいますが仕方ありません。
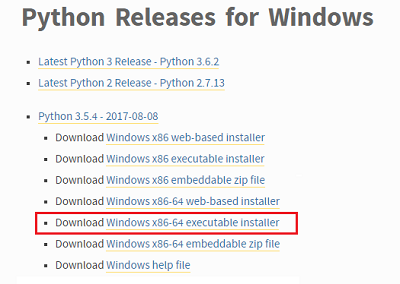
https://www.python.org/downloads/windows/
インストールが終わったら、環境変数にPythonへのパスを通しておきましょう。インストール場所は、Program Filesではないで注意が必要です。
%USERPROFILE%\AppData\Local\Programs\Python\Python35\
%USERPROFILE%\AppData\Local\Programs\Python\Python35\Scripts\
TensorFlow をインストールするのは簡単です。コマンドプロンプトから以下のコマンドを打つだけです。私のノートパソコンはGPUのような大層なものはないので、NonGPU版をインストールします。グラフ描画用の matplotlib もインストールしておきました。
C:\User\Taro>pip3 install tensorflow C:\User\Taro>pip3 install matplotlib
インストールされているか確認をするために動かしてみます。
C:\Users\Taro>python Python 3.5.4 (v3.5.4:3f56838, Aug 8 2017, 02:17:05) [MSC v.1900 64 bit (AMD64)] on win32 Type "help", "copyright", "credits" or "license" for more information. >>> import tensorflow as tf >>> hello = tf.constant('Hello, TensofFlow') >>> sess = tf.Session() 2017-08-29 23:51:36.686031: W C:\tf_jenkins\home\workspace\rel-win\M\windows\PY\35\tensorflow\core\platform\cpu_feature_guard.cc:45] The TensorFlow library wasn't compiled to use AVX instructions, but these are available on your machine and could speed up CPU computations. >>> print(sess.run(hello)) b'Hello, TensofFlow' >>>
なんか、変なメッセージが出ましたが動いたようです。今のままだと処理速度が遅いようですね。最適化するには、環境変数におまじないを追加するだけでよさそうです。以下のプログラムでメッセージが出なくなりました。
import os os.environ['TF_CPP_MIN_LOG_LEVEL']='2' import tensorflow as tf hello = tf.constant('Hello, TensofFlow') sess = tf.Session() print(sess.run(hello))
これで早くなったのかな?スクリプトが短すぎてよく分かりません。
TensorFlow を試してみるには、本家本元のこちらのサイトが一番分かりやすそうです。
Getting Started With TensorFlow
https://www.tensorflow.org/get_started/get_started
さて、環境も整ったので、Deep Learning を学習していこうかな。
(^^)/~

ゼロから作るDeep Learning ―Pythonで学ぶディープラーニングの理論と実装
- 作者: 斎藤 康毅
- 出版社/メーカー: オライリージャパン
- 発売日: 2016/09/24
- メディア: 単行本(ソフトカバー)

TensorFlow機械学習クックブック Pythonベースの活用レシピ60+ (impress top gear)
- 作者: Nick McClure
- 出版社/メーカー: インプレス
- 発売日: 2017/08/14
- メディア: 単行本(ソフトカバー)

初めてのディープラーニング --オープンソース"Caffe"による演習付き
- 作者: 武井 宏将
- 出版社/メーカー: リックテレコム
- 発売日: 2016/02/19
- メディア: 単行本(ソフトカバー)
Deep Learning を学ぶために Python をセットアップ [AI]
仕事で Deep Learning が必要になり、慌てて勉強をはじめています。同僚から勧められた教材を読み始めました。
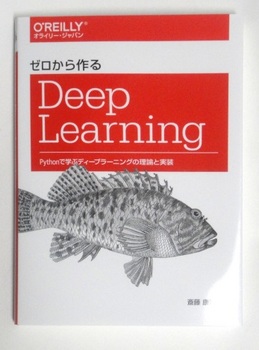
教材では Python を使うため、早速PCにインストールしました。(Raspberry Pi では最近なじみになっている Python ですが、PCにはインストールされてませんでした。^^;) 教材では Python3 を使っているので、Python 3.6.1 をダウンロードしました。
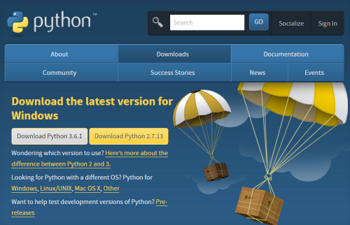
https://www.python.org/downloads/
インストーラーでインストールするのは簡単です。ただ、コマンドラインから使えるようにするには、プログラムのパスを設定する必要があります。いつも設定に右往左往するので、備忘録を兼ねて手順を書いておきました。
(1)コントロールパネルを開いて、”システムとセキュリティ”を選択します。
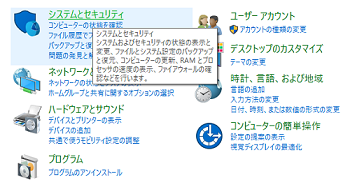
(3)システムを選択します。
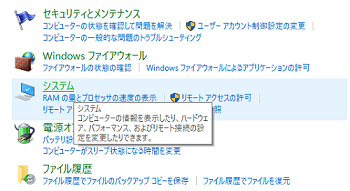
(4)システムの詳細設定を選択します。
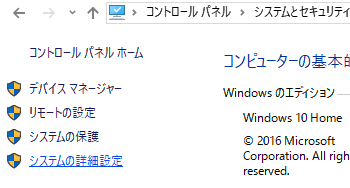
(5)システムプロパティの環境変数を選択。
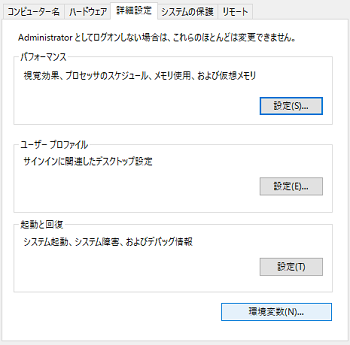
(6)ユーザー環境変数の"Path"に追加したいディレクトリパスを追加します。
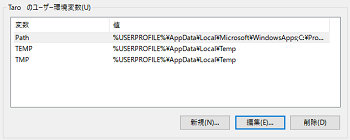
Python3 がインストールされているディレクトリは少し分かり難いところにあります。パスに以下の二つのディレクトリを追加しておけばコマンドラインからも Python を利用できます。
%USERPROFILE%\AppData\Local\Programs\Python\Python36-32
%USERPROFILE%\AppData\Local\Programs\Python\Python36-32\Scripts
次に教材で使うライブラリをインストールします。NumPy という数学用ライブラリと、関数描画用ライブラリ PyPlot をインストールします。
簡単な Python プログラムを作って動作確認をします。
コマンドラインから実行してみます。
すると綺麗な正弦関数のグラフが表示されました。
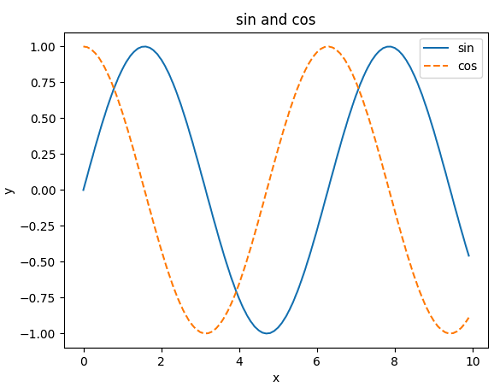
Deep Learning では画像を扱うことが多いので、PyPlot を使った画像表示用のサンプルプログラムもありました。
画像が表示されました。簡単ですねー。
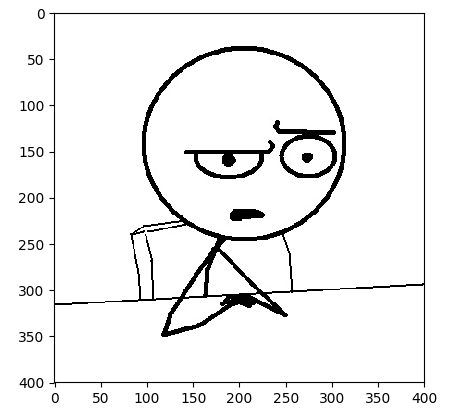
NumPy や PyPlot の使い方は、教材に詳しく記述されているので、必要な方は参照してください。特に NumPy は行列演算が簡単にできるので、Deep Learning に限らず数値計算には便利なライブラリです。
さて、これで準備ができました。いよいよ Deep Learning を勉強するかー。
σ(´ω`)


教材では Python を使うため、早速PCにインストールしました。(Raspberry Pi では最近なじみになっている Python ですが、PCにはインストールされてませんでした。^^;) 教材では Python3 を使っているので、Python 3.6.1 をダウンロードしました。
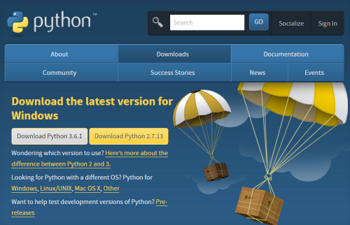
https://www.python.org/downloads/
インストーラーでインストールするのは簡単です。ただ、コマンドラインから使えるようにするには、プログラムのパスを設定する必要があります。いつも設定に右往左往するので、備忘録を兼ねて手順を書いておきました。
(1)コントロールパネルを開いて、”システムとセキュリティ”を選択します。
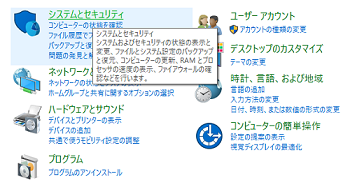
(3)システムを選択します。
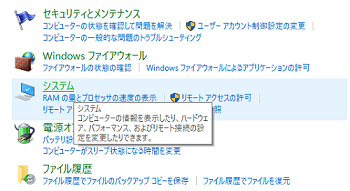
(4)システムの詳細設定を選択します。
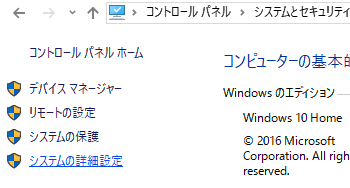
(5)システムプロパティの環境変数を選択。
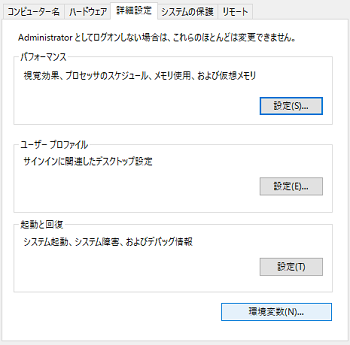
(6)ユーザー環境変数の"Path"に追加したいディレクトリパスを追加します。
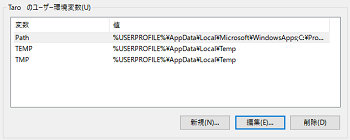
Python3 がインストールされているディレクトリは少し分かり難いところにあります。パスに以下の二つのディレクトリを追加しておけばコマンドラインからも Python を利用できます。
%USERPROFILE%\AppData\Local\Programs\Python\Python36-32
%USERPROFILE%\AppData\Local\Programs\Python\Python36-32\Scripts
次に教材で使うライブラリをインストールします。NumPy という数学用ライブラリと、関数描画用ライブラリ PyPlot をインストールします。
C:\User\Taro>pip install numpy C:\User\Taro>pip install matplotlib
簡単な Python プログラムを作って動作確認をします。
import numpy import matplotlib.pyplot as pyplot x = numpy.arange(0, 10, 0.1) y1 = numpy.sin(x) y2 = numpy.cos(x) pyplot.plot(x, y1, label="sin") pyplot.plot(x, y2, linestyle = "--", label="cos") pyplot.xlabel("x") pyplot.ylabel("y") pyplot.title('sin and cos') pyplot.legend() pyplot.show()
コマンドラインから実行してみます。
C:\User\Taro>python sample.py
すると綺麗な正弦関数のグラフが表示されました。
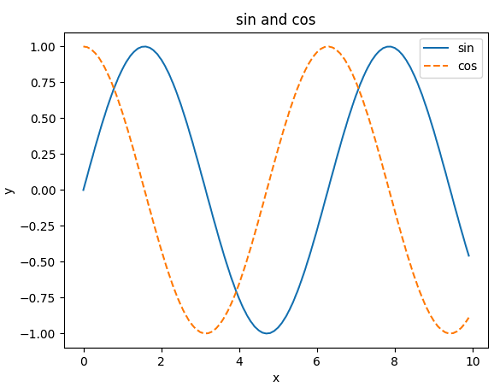
Deep Learning では画像を扱うことが多いので、PyPlot を使った画像表示用のサンプルプログラムもありました。
import matplotlib.pyplot as pyplot from matplotlib.image import imread img = imread('eyebrow_look.png') plt.imshow(img) plt.show()
画像が表示されました。簡単ですねー。
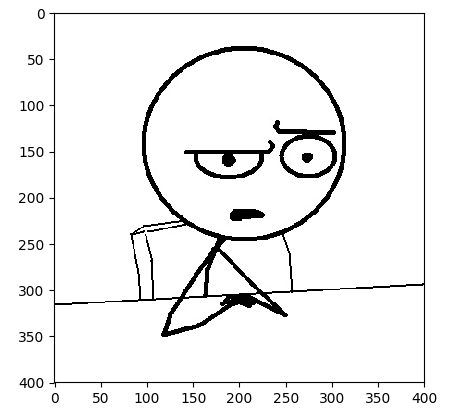
NumPy や PyPlot の使い方は、教材に詳しく記述されているので、必要な方は参照してください。特に NumPy は行列演算が簡単にできるので、Deep Learning に限らず数値計算には便利なライブラリです。
さて、これで準備ができました。いよいよ Deep Learning を勉強するかー。
σ(´ω`)

ゼロから作るDeep Learning ―Pythonで学ぶディープラーニングの理論と実装
- 作者: 斎藤 康毅
- 出版社/メーカー: オライリージャパン
- 発売日: 2016/09/24
- メディア: 単行本(ソフトカバー)

退屈なことはPythonにやらせよう ―ノンプログラマーにもできる自動化処理プログラミング
- 作者: Al Sweigart
- 出版社/メーカー: オライリージャパン
- 発売日: 2017/06/03
- メディア: 単行本(ソフトカバー)
Deep Learning のお勉強 [AI]
最近、猫も杓子もAIだーと騒がれていますが、会社にもじわじわとその波が・・・。ずっと気にはなっていたので、AI関連の仕事をしている会社の同僚から、よい入門書を紹介してもらいました。
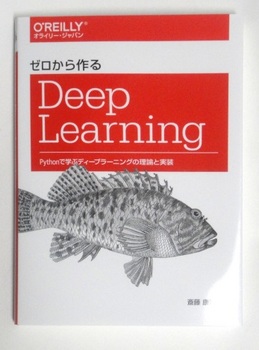
まだ斜め読みなのですが、遠い昔の学生時代にニューラルネットワークで画像診断ができないか研究していたこともあったので、意外と内容は分かります。
息子も興味を示していたので、これから少しずつ一緒に勉強していきたいと思います。ドローンにうまく活かせないかなーー。
(^_^)/~


まだ斜め読みなのですが、遠い昔の学生時代にニューラルネットワークで画像診断ができないか研究していたこともあったので、意外と内容は分かります。
息子も興味を示していたので、これから少しずつ一緒に勉強していきたいと思います。ドローンにうまく活かせないかなーー。
(^_^)/~

ゼロから作るDeep Learning ―Pythonで学ぶディープラーニングの理論と実装
- 作者: 斎藤 康毅
- 出版社/メーカー: オライリージャパン
- 発売日: 2016/09/24
- メディア: 単行本(ソフトカバー)

人工知能の作り方 ――「おもしろい」ゲームAIはいかにして動くのか
- 作者: 三宅 陽一郎
- 出版社/メーカー: 技術評論社
- 発売日: 2016/12/06
- メディア: 単行本(ソフトカバー)